Project Number: 071
Category: Emissions
This project sets out to develop improved knowledge on non-volatile particulate matter (nvPM) emissions to enable the development of soot-reduction technologies. A multi-scale hierarchical simulation strategy is proposed as a predictive tool for soot emissions from gas turbine engines. In gas turbines as the soot particles are generated, they can either oxidize completely or escape oxidation in the form of solid particles. A new multiscale approach will be established to predict soot formation in aircraft engines by integrating “first principle” simulations (used to account for the multi-scale physics of soot formation and transport) with large-eddy simulation (LES) that can be used to simulate turbulent flames in realistic engine combustors. We will explicitly target and isolate the layers of empiricisms that currently exist for example, in particle inception models, in the role of precursor species in nucleation, the sensitivity of predictions to particle shape and size distribution and the ad hoc coagulation/coalescence mechanisms. All of the tools that will be used already exist within the research team but a systematic coupling of these tools in a multi-scale, LES strategy has yet to be accomplished by anyone. The challenge here is to ensure high fidelity in predictions at the requisite time/length scales and then up-scale these effects into sub grid representation that can be used in a relatively cost-effective predictive simulations using LES.
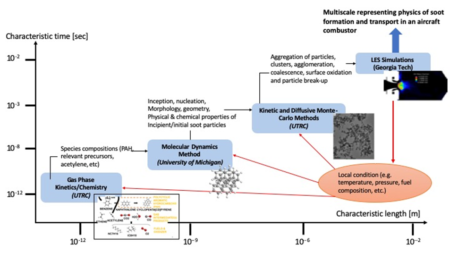
Outcomes
This project will advance the state-of-art in modeling soot formation and emission processes for aircraft engine combustors to enable innovative means to reduce these emissions. Use of real jet fuel chemistry will highlight the impact of fuel components and their reaction pathways on soot formation, and hence would be of direct relevance to legacy and next generation aircraft engines. Integrating these sub-models in LES will enable study of realistic gas turbine configurations. The proposed work addresses knowledge gaps, leveraging and building upon a long history of fundamental and modeling strategies at Georgia Institute of Technology, University of Michigan, and United Technology Research Center (UTRC).
Last Updated 4/12/2023
Annual Reports
Participants
Lead Investigators
Program Managers
Publications
- SPIN: A data-driven model to reduce large chemical reaction networks
- Predicting Aggregation Rates of Polycyclic Aromatics Through Machine Learning
- Elucidating the Polycyclic Aromatic Hydrocarbons Involved in Soot Inception
- A Machine Learning Framework to Predict the Aggregation of Polycyclic Aromatic Compounds
- Model Simulations of Soot Formation due to PAH Kinetics from Mixing Layers of Jet-A Fuels
- Exploring Soot Inception Rate with Stochastic Modelling and Machine Learning
- Uncertainty-based Weight Determination for Surrogate Optimization
- On the Importance of Species Selection for the Formulation of Fuel Surrogates
- Molecular Structures in Flames: A Comparison Between SNapS2 and Recent AFM Results
- Stochastic and Network Analysis of Polycyclic Aromatic Growth in a Coflow Diffusion Flame
- Oxidation of 2,6-dimethylheptane at Low Temperature: Kinetic Modeling and Experimental Study
- Reaction Pathways for the Formation of Five-membered Rings onto Polyaromatic Hydrocarbon Framework